Why Replicant exists?
Prior to mid-2000s, data management platforms belonged to one of the two broad categories, on-premise on-line transaction processing database systems (RDBMS) and on-premise decision support systems. It was a long era of isolated enterprise data warehouses (such as Teradata) curating information from on-line system of records (SQL based OLTP systems such as Oracle, DB2, Sybase, HP Nonstop) and of business intelligence software used to query such information. The process of curation gave rise to the concept of ETL (extract from system of records, transform into proper structures, and load into target data warehouses). ETL solutions were developed by inexperienced professionals, as a set of brittle offline scripts that would copy data out of the system of records periodically and infrequently. Enterprises got stuck with such a status quo because
most data sets were small enough in volume
They were generated with low velocity
Businesses did not demand real time insights on fresh data
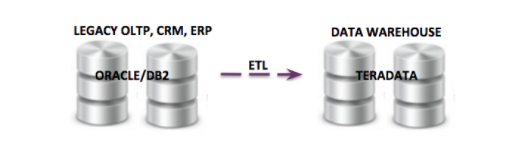
The last decade witnessed a rapid overhaul in the area of data management. With the advent of ubiquitous data sources resulting in unprecedented explosion in ingestion volumes, multiple data platforms had to evolve. Horizontally scalable and available NoSQL systems such as Cassandra and HBase evolved to handle high volume and velocity of online traffic with varying degrees of consistency and transactional requirements. Hadoop based systems evolved to handle batch analytics on high volumes of data. However, the same problems in the area of data movement prevailed. ETL processes still remained a pain with slow reaction to changing business demands.
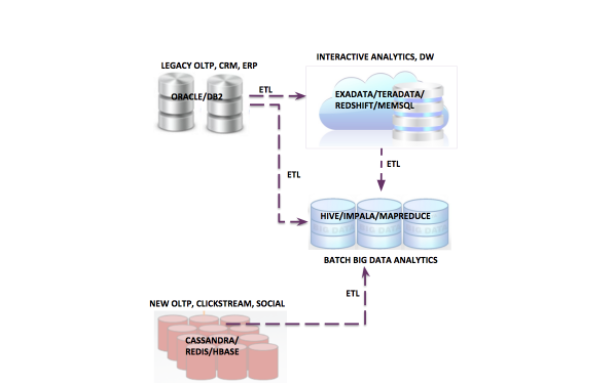
The whole NoSQL/Hadoop movement over the last 10 years have led to a fragmented data landscape with a plethora of data management solutions co-existing with the traditional ones, each serving some definite purpose, such as graph databases, time series databases, streams processors, etc. An enterprise customer today is plagued with the onus of variety. Moreover, deployment models have been evolving from being on-premise to moving to public cloud based ones.
As a group of professionals witnessing such rapid changes in data management landscape in terms of workloads and deployment models, we felt that it is the correct time to evolve the data movement landscape; to build an UNIVERSAL data movement solution that would meet the needs of today’s customers facing the carnage of data management solutions, from X to Y, from Y to Z, from on-premise to cloud; a solution that could handle variety, velocity, volume of today’s businesses in REAL TIME without compromising on data integrity and correctness, a solution that had to provide consumer style manageability to enterprise environments.
The result: We built the power station while everyone else is busy re-building the light bulb. Here is a teaser. We will talk more about it in subsequent blogs.

Comments